Oct 31, 2024
Huck researchers reflect on the 2024 Nobel Prize in Chemistry
This month, the Nobel Prize in Chemistry was awarded to three scientists credited with historic breakthroughs surrounding proteins and their structures. Three Huck researchers working on similar challenges chime in with their thoughts.
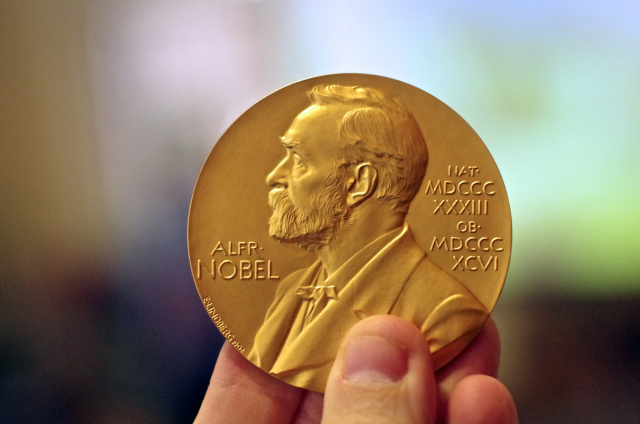
The 2024 Nobel Prize in Chemistry was awarded to a trio of scientists who, as the Royal Swedish Academy of Sciences put it, “cracked the code for proteins’ amazing structures.” Half the prize went to David Baker at U of Washington, for computationally designing entirely new kinds of proteins. Demis Hassabis and John M. Jumper, both from Google DeepMind, London, shared the other half of the prize for developing the AI-powered AlphaFold Protein Structure Database to predict protein structures from amino acid sequences.
“Proteins are workers of life, executing almost all aspects of cellular and extracellular actions. Their structures are intimately related to their functions, which has inspired a communal quest to find rules that relate amino acid protein sequence to function. It turns out that Nature has imprinted some of the rules in protein evolution. Human efforts have resulted in remarkable means to predict protein structure from sequence using knowledge derived from evolutionarily related proteins. With the emergence of deep machine learning techniques, these patterns became much more apparent, and now the structure prediction problem has been solved thanks to Google’s DeepMind crew, led by Demis Hassabis and John M. Jumper.
Protein design is an inverse problem: if we know the structure of a hypothetical, can we accurately predict the sequence of such protein? Physicist Richard Feynman, who famously said: "What I cannot create, I do not understand." In a sense, protein design problem is deeply rooted in our ability to predict structure. David Baker together with Brian Kuhlman (UNC Chapel Hill) were the first ones to create a completely new protein Top7 that nobody has seen in Nature in 2003. Although monumental work was done prior to them by Bill DeGrado and Stephen Mayo among others, the creation of Top7 was a landmark achievement that spurred in rapid growth in molecular engineering techniques.
My laboratory has developed own means for protein design because for some time we have collaborated with David Baker, Brian Kulman, Stephen Mayo, and others. This collaboration has allowed us to design novel vaccines for HIV and COVID, among many other proteins that are regulatable by light or drugs. Although none were commercialized, the success of our protein design is largely attributed to these collaborations.” – Nikolay Dokholyan, G. Thomas Passananti Professor of Pharmacology; Professor of Biochemistry and Molecular Biology
“In any domain in which large, high quality representative training data are available, modern machine learning algorithms offer unprecedented opportunities for extracting useful insights from data. Protein structure prediction is no exception.
The Google DeepMind team led by Hassabis and Jumper trained a massive deep neural network on all of the known structures in the protein Data Bank to predict protein structure from amino acid sequence. My group has used similar machine learning approaches for many years, on smaller data sets to predict protein-protein and protein-RNA interactions. That said, there is still a great deal we do not understand about how proteins fold or how they interact with other proteins, RNA, etc.
A promising direction is to develop interpretable machine learning models - either models that are interpretable by design or models that can be interpreted with the help of algorithms to gain physical insights into what they have learned. Ongoing work in the Penn State Center for Artificial Intelligence Foundations and Scientific Applications (CENSAI) and NSF National Synthesis Center for Emergence in the Molecular and Cellular Sciences led by Ed O'Brien of Chemistry is aimed at gaining such biological insights by leveraging recent breakthroughs in machine learning.” – Vasant Honavar, Huck Chair in Biomedical Data Sciences and AI; Professor and Edward Frymoyer Chair of Information Sciences and Technology
“My research group focuses on understanding gene expression, a fundamental process where the genetic code is used in cells. With support from the National Institutes of Health (NIH), we have made important advances in understanding how RNA polymerase (RNAP) carries out gene expression in various organisms, including bacteria, archaea, and viruses. We use advanced techniques available at the Huck X-ray Crystallography and Cryo-Electron Microscopy (Cryo-EM) Facilities to capture 3D images of RNAP at different stages of transcription.
Before we start experiments, we first need to investigate if our target macromolecule is suitable for either X-ray or cryo-EM methods. In the past, this required a lot of time and effort to assess the stability and behavior of macromolecules under different conditions using biochemical and biophysical methods.
The revolutionary thing about AlphaFold is that it can instantly predict the 3D structure of molecules just from their amino acid sequences. This has saved us a lot of time in figuring out whether our target macromolecule is suitable for structure determination experiments. The latest version of AlphaFold3 can now predict the structure of complexes that include proteins, DNA, and RNA, making it easier to plan research for investigating the RNAP transcription complexes and their interactions with transcription factors. By determining these 3D structures experimentally by cryo-EM, we can advance our projects such as discovering new transcriptional regulatory mechanisms/functions and developing drugs that target transcription.
One of the best features of AlphaFold is that it’s easy to use, even for people who don’t have an experience and knowledge with computer-based structure prediction. In our lab, undergraduate researchers can now contribute to several research projects by using AlphaFold3 to predict macromolecular structures thus propose experimental conditions for cryo-EM study. This means that even students who have limited time in the lab can still play an active role in our research project supported by NIH.” – Katsuhiko Murakami, Faculty Director of the Cryo-Electron Microscopy Core Facility; Professor of Biochemistry and Molecular Biology